By Nirmal John
AI Water Consumption: The Hidden Environmental Cost of Artificial Intelligence
Wednesday April 2, 2025
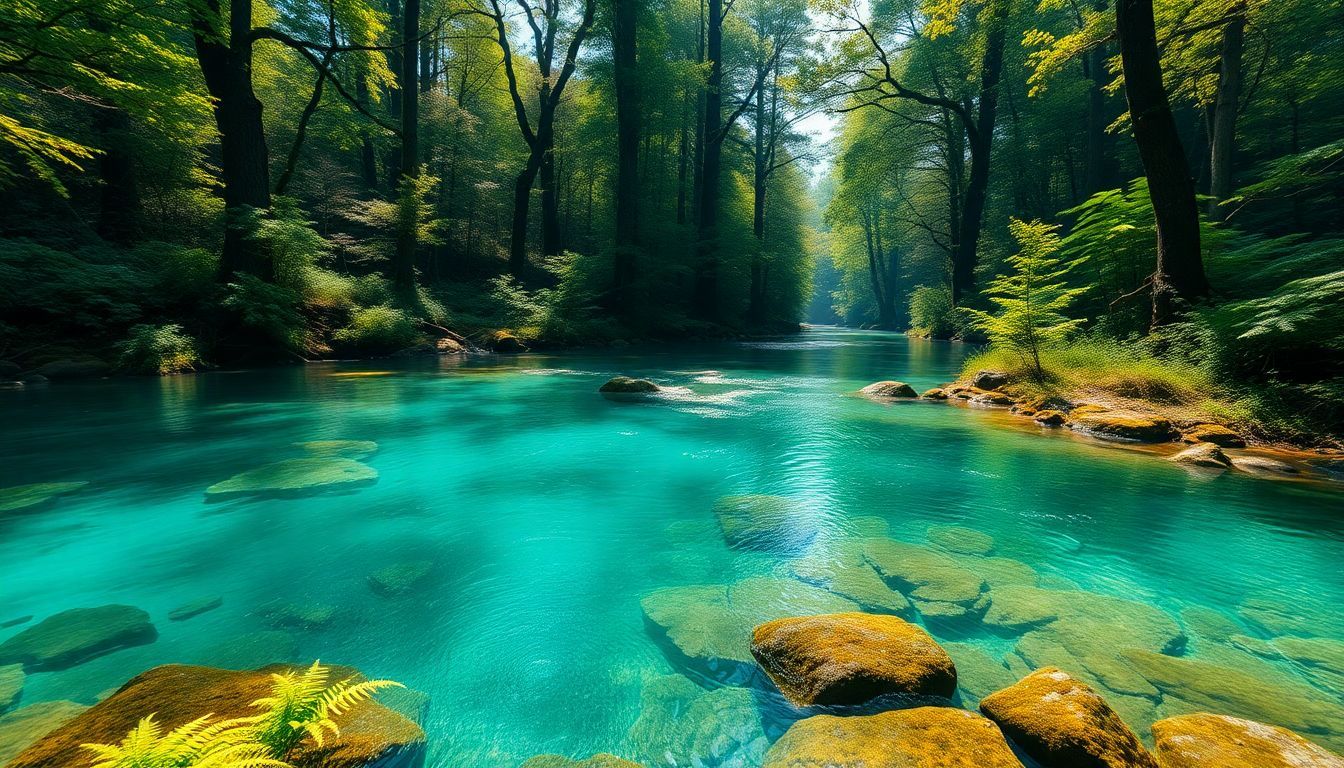
AI Water Consumption: The Hidden Environmental Cost of Artificial Intelligence
Introduction: AI’s Growing Thirst for Water Resources
Did you know that global water demand could outstrip supply by 40% in just a few years? The AI environmental impact on water is an often-overlooked consequence of artificial intelligence’s rapid integration into our lives. From smart home devices to autonomous vehicles, AI is revolutionizing how we live and work. However, beneath this technological transformation lies a critical environmental challenge: the massive water consumption required to cool data centers that power AI systems.
The computational power driving AI systems requires massive data centers that consume extraordinary amounts of water for cooling. This escalating water usage presents a growing environmental challenge that deserves immediate attention as AI continues its exponential growth trajectory.
While artificial intelligence offers tremendous potential for solving complex problems and improving efficiency across industries, its environmental footprint—particularly regarding water resources—raises serious sustainability questions. The relationship between AI advancement and water conservation represents one of the most crucial environmental balancing acts of our digital age.
The Growing Water Demands of AI Systems
AI systems require substantial processing power to function effectively. This processing capacity comes primarily from sprawling data centers filled with high-performance servers and computing infrastructure. These facilities not only consume enormous amounts of electricity but also rely heavily on water resources for critical cooling operations.
The connection between AI’s environmental impact on water consumption and computational power is direct and concerning. As AI models become more sophisticated and processing demands increase, the corresponding water requirements grow proportionally. This relationship creates a sustainability challenge that the tech industry must address proactively rather than reactively.
Recent research from the University of California estimates that training a single large language model can indirectly consume up to 500,000 gallons of water—equivalent to the amount needed to manufacture 56 cars or fill five residential swimming pools. This water footprint occurs before the AI system even begins its operational lifecycle, highlighting the resource-intensive nature of artificial intelligence development.
Data Centers: The Water-Intensive Infrastructure Behind AI
Data centers represent the physical backbone of the AI revolution. These massive facilities house thousands of servers working simultaneously to power AI operations—from simple voice assistants to complex machine learning systems. The heat generated by these servers creates a substantial cooling challenge that often relies heavily on water-based solutions.
A single large-scale data center can consume between 3-5 million gallons of water daily for cooling purposes—roughly equivalent to the water usage of a town with 30,000-50,000 residents. This staggering level of AI water consumption places significant pressure on local water resources, especially in regions already experiencing water stress.
While some leading tech companies are implementing water conservation technologies in their newest facilities, many existing data centers continue to operate with less efficient cooling systems. The industry’s rapid expansion means that even as efficiency improves in newer facilities, the aggregate water demand continues to rise due to the growing number and scale of data centers worldwide.
Water usage in data centers varies significantly based on factors like facility design, geographic location, and the specific cooling technologies employed. Direct water cooling systems typically use the most water but offer greater energy efficiency, creating a complex sustainability tradeoff that facility managers must navigate carefully.
The Training Thirst: Water Footprint of AI Model Development
The development and training of sophisticated AI models represents one of the most water-intensive aspects of artificial intelligence. Training a single large language model can require thousands of high-performance processors running continuously for weeks, consuming massive amounts of electricity in the process.
This energy often comes from power plants that themselves require substantial water resources for cooling and operation. Consequently, the AI environmental impact on water extends beyond direct consumption, as the indirect water usage associated with model training can be enormous even before accounting for operational demands once the model is deployed.
The computational requirements for training advanced AI models have been doubling approximately every 3.4 months—a rate far exceeding Moore’s Law. This exponential growth in processing needs translates directly to increased water demands, creating an unsustainable trajectory if not addressed through more efficient algorithms and sustainable energy sources.
Leading AI research lab DeepMind has estimated that training their largest models can indirectly consume enough water to sustain a small community for several days. As models continue to grow in size and complexity to achieve greater capabilities, this water footprint expands accordingly, underscoring the environmental cost of AI water consumption and the urgent need for sustainable innovation.
AI-Powered Water Management: A Sustainability Paradox
Ironically, artificial intelligence offers promising applications for water conservation and management. AI systems can optimize irrigation schedules, detect infrastructure leaks, predict maintenance needs, and improve overall water distribution efficiency—potentially saving billions of gallons annually across various industries.
However, this creates a fundamental paradox: the very systems designed to enhance water efficiency themselves consume substantial water resources. The critical question becomes whether the water savings generated by AI applications can offset the impact on AI water consumption required to develop and operate these systems.
Early research suggests that well-designed AI water management systems can generate net-positive water impacts, potentially saving 10-15 times more water than they consume. However, these benefits typically occur in different geographic locations than the water usage, creating regional disparities that complicate the sustainability equation.
For AI to truly contribute to water sustainability, the industry must simultaneously pursue two objectives: maximizing the water-saving potential of AI applications while minimizing the water footprint of AI infrastructure and operations.
Quantifying AI’s Water Footprint: Understanding the Scale
Accurately measuring AI water consumption presents significant challenges due to the distributed nature of AI infrastructure and the lack of standardized reporting requirements. However, emerging research is beginning to quantify this impact more precisely.
A 2023 study published in the Journal of Environmental Science and Technology estimated that the global AI industry currently consumes approximately 12-14 billion gallons of water annually—a figure projected to double by 2027 if current growth trajectories continue without significant efficiency improvements.
This water usage includes both direct consumption (water used directly for cooling) and indirect consumption (water used in electricity generation). Understanding these different components provides a more comprehensive picture of AI’s total water footprint and identifies priority areas for conservation efforts.
Estimating Water Consumption per AI Task
Breaking down AI water consumption to the level of individual tasks helps illustrate the cumulative impact of everyday AI usage. While a single search query or chatbot interaction uses a relatively small amount of water, the scale of these operations creates substantial aggregate demand.
Research from the MIT Technology Review suggests that:
- A typical AI-powered search query indirectly consumes approximately 0.1-0.3 liters of water
- A 10-minute interaction with an AI assistant uses roughly 0.5-1.5 liters
- Training a custom enterprise AI model can consume 100,000-500,000 liters
These figures highlight how seemingly insignificant individual actions accumulate into substantial resource demands when multiplied across billions of daily interactions worldwide. Understanding this relationship between scale and impact is crucial for developing appropriate sustainability strategies.
Regional Variations in AI Water Impact
The environmental impact of AI water consumption varies dramatically based on geographic location, available water resources, and local energy infrastructure. Data centers located in water-stressed regions naturally create more significant environmental pressure than those in water-abundant areas.
Similarly, the source of electricity powering AI operations substantially affects water usage. Data centers relying on coal or nuclear power indirectly consume significantly more water than those powered by solar, wind, or other renewable sources that require minimal water inputs.
These regional variations create both challenges and opportunities. While they complicate universal sustainability standards, they also allow for strategic facility placement and energy sourcing to minimize water impacts in the most vulnerable regions.
Environmental Consequences of Unchecked AI Water Use
Without appropriate conservation measures, the growing AI water consumption trend threatens to exacerbate existing environmental challenges. Water scarcity already affects over 40% of the global population, and climate change continues to disrupt precipitation patterns and water availability worldwide.
In this context, the water demands of the expanding AI industry risk intensifying regional water stress, contributing to ecosystem degradation, and potentially creating conflicts between technology development and community water needs.
Water Scarcity and Resource Competition
In water-limited regions, data centers often compete directly with agriculture, manufacturing, and residential needs for available water resources. This competition can drive up water prices, complicate resource allocation decisions, and potentially contribute to water inequity issues.
For instance, in drought-prone areas of the western United States, large data center developments have faced community opposition due to concerns about water usage. These conflicts highlight the importance of transparent water management practices and community engagement in facility planning.
Responsible AI water consumption strategies must include careful consideration of local water availability, community impacts, and fair resource allocation principles to avoid exacerbating existing inequities or creating new sustainability challenges.
Impact on Aquatic Ecosystems and Biodiversity
Beyond simple resource competition, the AI environmental impact on water extends to aquatic ecosystems through various mechanisms. Water withdrawal from natural sources can alter flow patterns and habitat availability, while thermal pollution from discharged cooling water can disrupt sensitive aquatic environments.
Data centers using “once-through” cooling systems release warmer water back into local waterways, potentially altering oxygen levels and affecting temperature-sensitive species. These ecological impacts highlight how AI water consumption affects ecosystems beyond simple resource depletion.
Comprehensive environmental assessment and monitoring programs are essential to understand and mitigate these effects, particularly as data center development accelerates in new geographic regions with varying ecological sensitivities.
Strategies for Water-Sustainable AI Development
Despite these challenges, numerous promising strategies exist to reduce AI water consumption while maintaining technological progress. The AI industry has both the resources and innovation capacity to dramatically improve its water sustainability profile through targeted investments and operational changes.
Optimizing Data Center Cooling Technologies
Cooling technology innovations represent one of the most direct approaches to reducing water usage in AI infrastructure. Several alternatives to traditional water-intensive cooling methods show promising efficiency improvements:
- Air-side economization uses external air for cooling when climate conditions permit, potentially reducing water usage by 80-90% compared to traditional cooling towers
- Closed-loop cooling systems recirculate and reuse water, dramatically reducing consumption compared to once-through systems
- Immersion cooling submerges computing components in non-conductive fluids, eliminating water needs while improving energy efficiency
- Direct-to-chip cooling targets heat removal precisely where it’s generated, improving efficiency and reducing resource requirements
Microsoft’s Project Natick demonstrated another innovative approach by placing data centers underwater, using the natural cooling properties of the ocean while eliminating freshwater consumption entirely. While not universally applicable, such creative solutions illustrate the potential for dramatic improvements in water efficiency.
Developing Water-Efficient AI Algorithms and Models
Computational efficiency improvements can significantly reduce the resources required for AI operations. More efficient algorithms and model architectures directly translate to lower energy consumption and, consequently, reduced AI water consumption.
Research into model distillation techniques—methods for creating smaller, more efficient models that maintain most capabilities of larger ones—shows particular promise. These “lightweight” AI systems can perform nearly as well as their larger counterparts while requiring a fraction of the computational resources.
Similarly, specialized AI hardware designed for efficiency rather than raw performance can dramatically reduce resource requirements for specific applications. These purpose-built chips optimize power consumption and heat generation, directly impacting water needs for cooling.
Promoting Renewable Energy Sources for AI Operations
The water intensity of different electricity sources varies dramatically. Coal and nuclear power plants typically use 20-60 gallons of water per kilowatt-hour produced, while solar photovoltaic and wind power require virtually no water for operation.
Transitioning AI infrastructure to renewable energy sources therefore represents one of the most effective strategies for reducing indirect AI water consumption. Many leading tech companies have already made significant commitments to renewable energy, but accelerating this transition across the entire industry remains crucial.
Google’s AI facilities, for example, now operate with 90% carbon-free energy on an annual basis, significantly reducing their water footprint compared to fossil fuel alternatives. These initiatives demonstrate that environmentally responsible AI operation is both technically feasible and economically viable.
Policy and Regulation for Water-Conscious AI Development
While voluntary industry initiatives play an important role in promoting sustainability, comprehensive policy frameworks will likely be necessary to ensure responsible AI water consumption practices across the entire sector.
Effective regulation might include:
- Mandatory water usage reporting and disclosure requirements
- Water efficiency standards for new data center developments
- Incentives for implementing water conservation technologies
- Consideration of local water availability in facility permitting decisions
- Research funding for water-efficient computing technologies
Several jurisdictions have already begun implementing such measures. For instance, the European Union’s sustainable data center initiatives aim to make data centers climate-neutral and highly resource-efficient by 2030, including specific targets for water conservation.
Conclusion: Balancing Innovation and Water Stewardship
Artificial intelligence offers tremendous potential to transform our world, but the AI environmental impact on water presents a significant sustainability challenge that must be addressed proactively. The growing water demands of AI infrastructure risk exacerbating existing resource pressures and creating new environmental challenges if not managed responsibly.
Fortunately, numerous technical, operational, and policy solutions exist to dramatically improve the water efficiency of AI systems. By implementing cooling technology innovations, developing more efficient algorithms, transitioning to renewable energy, and establishing appropriate regulatory frameworks, the AI industry can significantly reduce its environmental impact.
The path forward requires commitment from technology companies, researchers, policymakers, and consumers to prioritize sustainability alongside innovation. With thoughtful development practices and continued innovation in resource efficiency, AI can fulfill its transformative potential while mitigating its impact on global water consumption.
By advocating for sustainable AI practices and supporting organizations that prioritize environmental responsibility, we can collectively ensure that artificial intelligence becomes a force for positive change without imposing unacceptable costs on our planet’s precious water resources.
About the author
Recent articles
Quad Core Dedicated Servers
Interested in Quad Core Dedicated Servers? View our inventory. What is a quad core dedicated...
Read More8 Core Dedicated Servers
For website owners looking to eliminate their hardware bottlenecks, the massive power capabilities of an...
Read MoreHow Unmetered Servers Can Help Businesses Grow
If you have a business website that is growing and expanding, it is essential that...
Read More